“Hey Smart ChatBot, I have a 10-hour layover in London. Could you please suggest what to do during this time?”
“No, I’m not interested in seeing the Changing of the Guard ceremony.”
“I wanna check out Shoreditch and grab some lunch at the Borough market.”
“Please suggest the fastest route considering I’ll need short-term luggage storage.”
“No, come on, I didn’t ask about the costs! I need to know the fastest route… Can you show me it on a map?”
[Bot shows a confusing, static map of the route I’d need to take.]
“Nevermind, I’ll just check Google Maps.”
This was the real-life chat I had as I was heading to the AWS GenAI Analyst Summit in Seattle last week.
I probably would have gotten better suggestions about things to do in London from a human.
If it had been a human personal assistant, they’d have known me already; and we could have figured everything out in a 5-minute phone call, personalizing my experience and sparing me the couple of hours spent chatting with a bot instead of catching up on work at the airport.
In short, GenAI provides great support but never seems to get the job done!
Nonetheless, the speed of development and adoption of the technology across industries has been unprecedented. And this was a key area of focus at the GenAI AWS Analyst Summit—which I attended the following day, after arriving in Seattle.
Despite enthusiasm among IT leaders and their desire to reap the benefits of GenAI, they often face roadblocks when it comes to management and adoption of this new culture: While CIOs may be excited to try out new GenAI tools, CFOs are hesitant to approve budgets, and CEOs and COOs want to see clear ROI and tangible value for their organizations.
Key Barriers to Adoption of GenAI
Compared to the cloud and especially IaaS—which have brought about seismic shifts, fundamentally altering enterprises’ core IT hardware infrastructure—GenAI is still in its infancy and is primarily a software advancement.
This difference implies a faster cycle of change, making the technology potentially ripe for rapid adoption and market saturation. But this accelerated adoption also brings with it challenges, such as the need for a robust implementation strategy, requiring significant investments and therefore greater risk.
This is in stark contrast to the early days of the Amazon cloud, when companies would “start small,” with just a few Amazon EC2 instances.
But with GenAI, we’re seeing “small” experiments quickly spiral into resource-intensive endeavors. This introduces complexities when attempting to select the appropriate tools and models, as well as customizing and training those models effectively on costly cloud resources.
I’ve seen companies fall into this trap. If you’re looking to avoid this, I recommend reading this GenAI cost optimization blog from AWS, which provides guidance on how to get started.
Yet another issue companies are facing with GenAI is that it may be relatively easy to pilot a smart siloed application, but scaling it to meet cross-organizational needs is complex and risky.
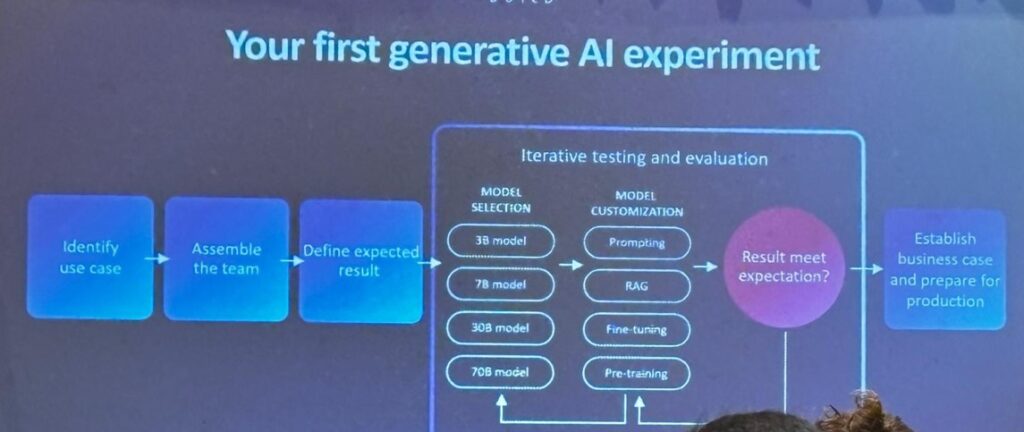
On top of that, IT organizations are still struggling with their data management, and GenAI hallucinations (when AI generates plausible but incorrect or nonsensical responses) certainly haven’t helped here. If your results aren’t reliable, business users might reconsider their investment. Because nothing is more important than trust, right?
Enterprise Adoption and Implementation of GenAI
According to AWS, in 2024, IT organizations moved from POCs to running GenAI in production. According to Gartner, however, this doesn’t seem to be the case:
“43% of CEOs have started investing in GenAI. An additional 45% are planning to do so within the next year. However, 90% of organizations are still in the early stages of GenAI maturity, either running proofs-of-concept or developing capabilities in isolated pockets of their business, which could be troubling for a few reasons.”
Speaking at the GenAI Summit was Veteran CIO, Enterprise Strategist and CxO Advisor at AWS Tom Godden. Though his talk was pretty high-level—and I’d say, even a bit fluffy—he provided some interesting perspectives.
When discussing how CIOs can navigate the GenAI landscape, he broke it down into six stages, as shown below:
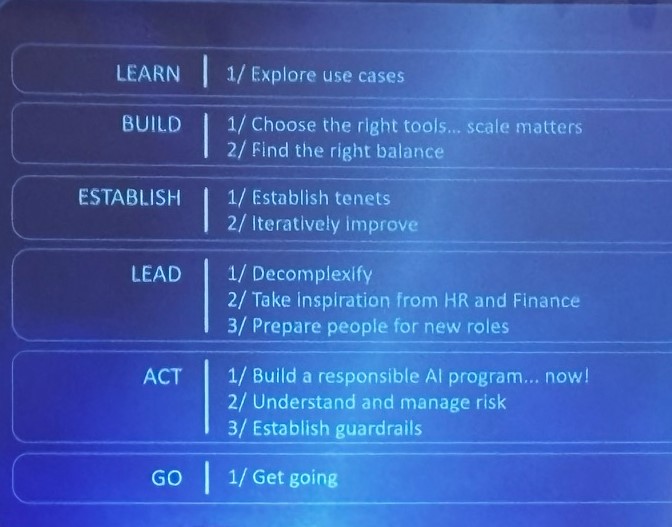
In this regard, I’d argue that smart adoption isn’t linear nor is it a step-by-step process. Rather, IT organizations should be distributed to push for bottom-up technology adoption and to empower young developers and newly recruited marketers to start by using whatever AI tools they prefer in order to streamline and simplify their workflows.
In the early days of AWS, Amazon EC2 offered a great parallel, where low-cost access democratized compute power and led to widespread adoption.
Similarly, GenAI has the potential to drive transformation. But unlike with hardware, GenAI software poses multiple challenges, such as uncontrolled fragmentation across teams and functions that can limit its impact and value.
Nonetheless, top-down adoption might take precedence. And this should be initiated by enterprise R&D and IT executives.
Another important point to consider is the implementation of GenAI within organizations. While R&D and IT teams may initiate GenAI projects from the ground up, as already mentioned, these efforts often remain siloed and disconnected from broader business goals. This, of course, can hinder its overall success and scalability.
Moreover, a structured adoption strategy, Godden highlighted, requires separation between centralized and decentralized duties (see image below).
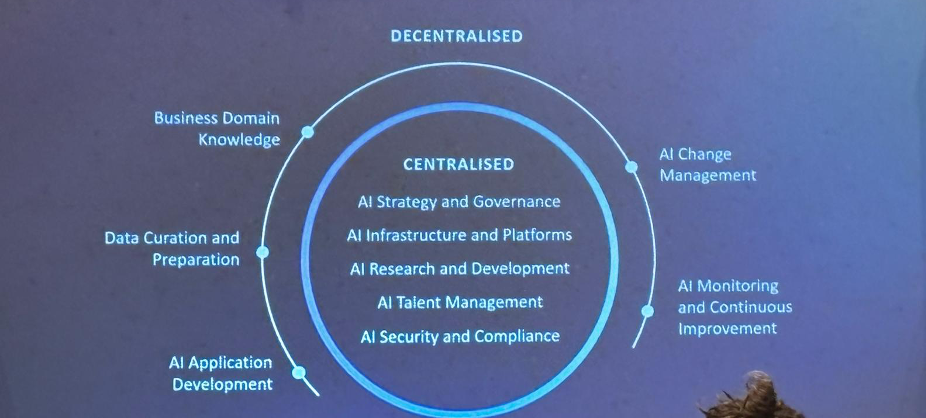
Furthermore, the shortage of GenAI experts presents yet another significant challenge. But instead of focusing solely on recruitment, IT and R&D leaders should prioritize reskilling and upskilling their existing teams. Not only will such efforts help create today’s new GenAI leaders; but more importantly, this will enable developers to adopt a GenAI-first mindset and will augment the applications and responsibilities they are already handling.
Last but not least is the data, which is what makes AI a “living creature.”
In this vein, Godden recommended implementing a data management strategy that treats data as a product, the ongoing enhancement of data repositories through integrations, and offering greater flexibility when it comes to access (I’ll be delving deeper into this topic in a separate blog post.).
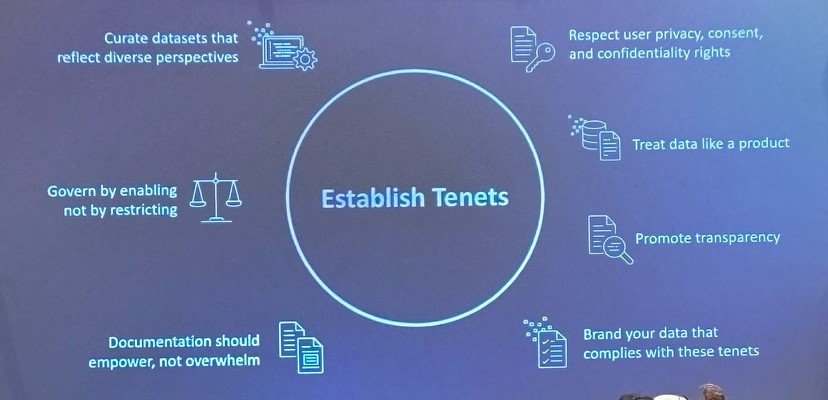
The AWS GenAI Offering
At the GenAI Analyst Summit, AWS showcased its many advancements in the field, highlighting Amazon SageMaker and Amazon Bedrock, (which IOD’s team of AI researchers is currently testing—stay tuned for our upcoming blog posts on the topic). Notably, over the past year, AWS has introduced hundreds of updates and launches related to these tools, emphasizing their commitment to staying ahead in the AI space.
AWS’s infrastructure is another critical factor in its dominance of the AI sector. Amazon SageMaker and Amazon Bedrock position AWS as an industry leader. This is especially due to their focus on hardware advancements through AWS Graviton and AWS Inferentia chips, which I’m always proud to mention were developed by the talented Israeli team Annapurna, which was acquired by AWS (Honestly though, I’m still more enthusiastic about what AWS did with the AWS Nitro chips!).
The Summit also highlighted some very interesting advanced Amazon Bedrock features to be released later this year.
The Infrastructure Advantage
The above developments underline AWS’s clear intent to create a robust revenue model for generative AI that is closely tied to its hardware capabilities.
Nevertheless, AWS still needs to differentiate itself from competitors like Microsoft Azure, especially as both platforms offer access to prominent AI models from OpenAI and Anthropic. AWS’s strategic collaborations and infrastructure will play a pivotal role in maintaining its competitive edge.
The AWS team reassured me that my concerns about AWS Q following my experience trying it out would be addressed at AWS re:Invent 2024 in December, where we can expect some important announcements about the service.
Looking Ahead: Multi-Agents
In between sessions, I had the opportunity to network with fellow bloggers and analysts. I had some interesting conversations, but one topic that came up that has intrigued me is the notion of multi-agent systems (MAS). These systems are composed of multiple autonomous agents that interact to achieve individual or collective goals (like having several team members).
These agents can be designed to work together or to compete with each other, depending on the application. This allows for dynamic interactions that can boost task performance such as resource management and data analysis.This will surely lead to new initiatives and levels of efficiency, improving ROI for enterprise initiatives, particularly in more sophisticated AI environments. Hopefully, this will finally make GenAI a tool that can get the job done for me! 🙂
Aside from the summit itself, it was great visiting the vibrant city of Seattle again. AWS invited us to an NFL game between the Seattle Seahawks and San Francisco 49ers, hosted us at a nice suite at Lumen Stadium, and also organized a fun cocktail night for the analysts at the Space Needle, which offered a stunning view of the city.
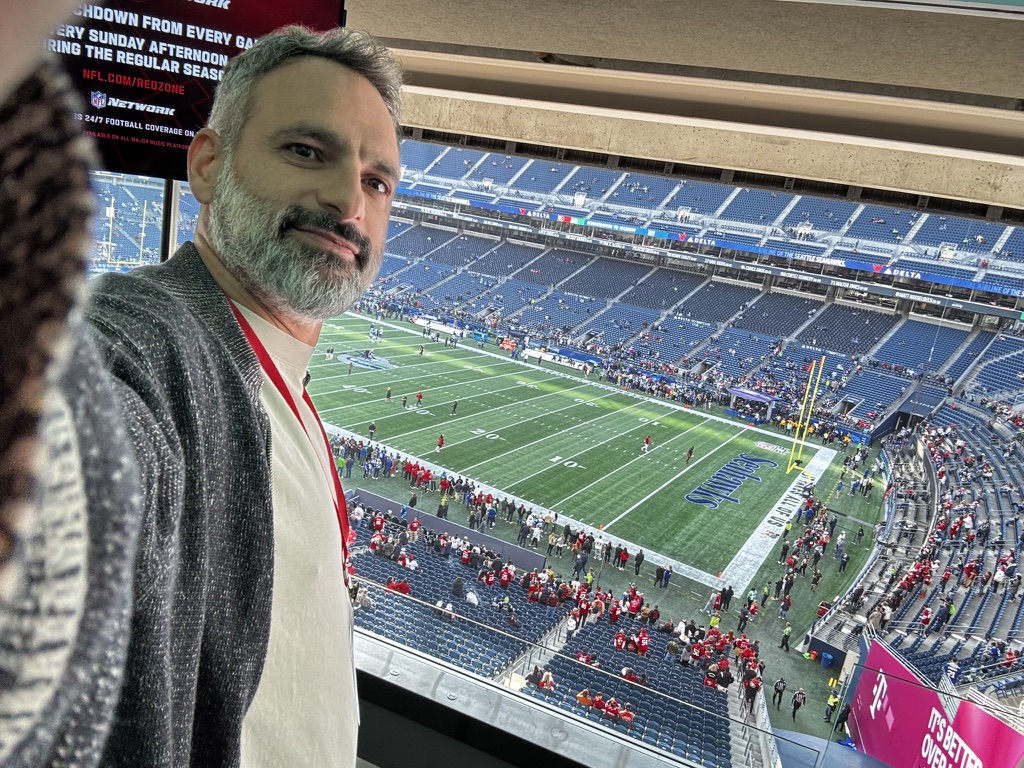
I also had some time to explore the city, strolling through the new Overlook Walk on the waterfront, the famous Seattle Pike market, and visiting the first Starbucks.
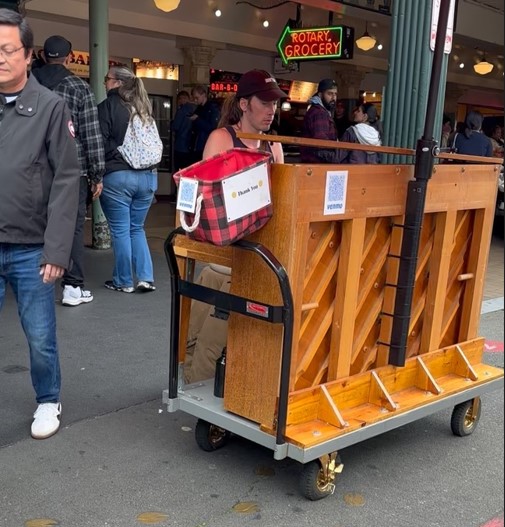
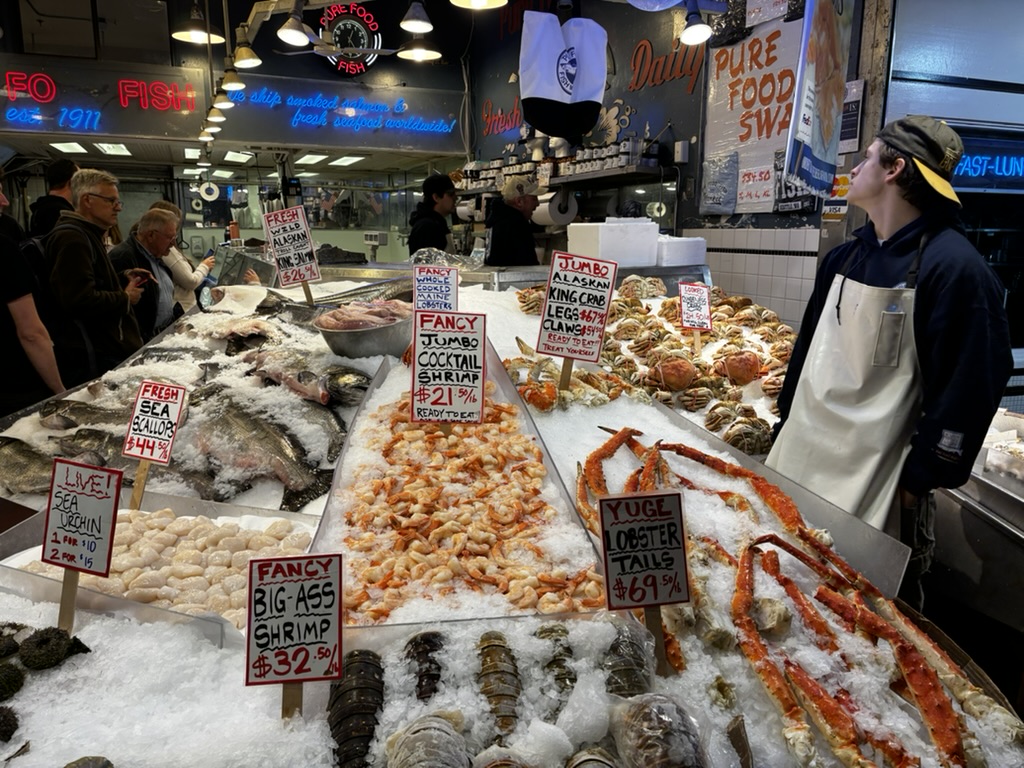
Next Stop: Vegas for the AWS re:Invent 2024!